Articles
Hereafter you can find the list of articles proposed for this class and the link to the pdfs.
Please add your name in the following file to pick an article and enter your github username.
Note 1: this work can be done in teams (maximum 3 students).
Note 2: an article can only be chosen by 1 team.
Tips for Latex
To activate latex writting you need to add this snippet of code (at the end or begining of the post)
code.has-jax {font: inherit; font-size: 100%; background: inherit; border: inherit;}
</style>
<script type="text/x-mathjax-config">
MathJax.Hub.Config({
tex2jax: {
inlineMath: [['$','$'], ['\\(','\\)']],
skipTags: ['script', 'noscript', 'style', 'textarea', 'pre'] // removed 'code' entry
}
});
MathJax.Hub.Queue(function() {
var all = MathJax.Hub.getAllJax(), i;
for(i = 0; i < all.length; i += 1) {
all[i].SourceElement().parentNode.className += ' has-jax';
}
});
</script>
<script type="text/javascript" src="https://cdnjs.cloudflare.com/ajax/libs/mathjax/2.7.4/MathJax.js?config=TeX-AMS_HTML-full"></script>
Tips for images
You can add images to the blogpost. To this end create a folder for your blogpost within the content/images
folder.
Then to add them in the blog post, you have two options.
Html
<p align="center">
<img src="/images/ip-logo.png" alt="ip paris logo">
</p>
Markdown
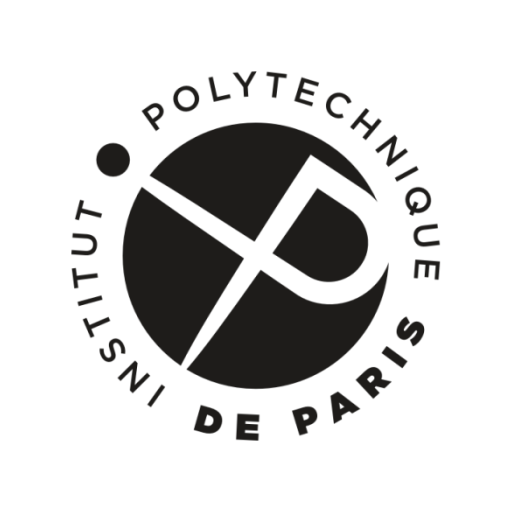